Master Award in
Deep Learning
Master Award could transfer 20 credits and full tuition fees to Master’s programs by SIMI and University Partners.
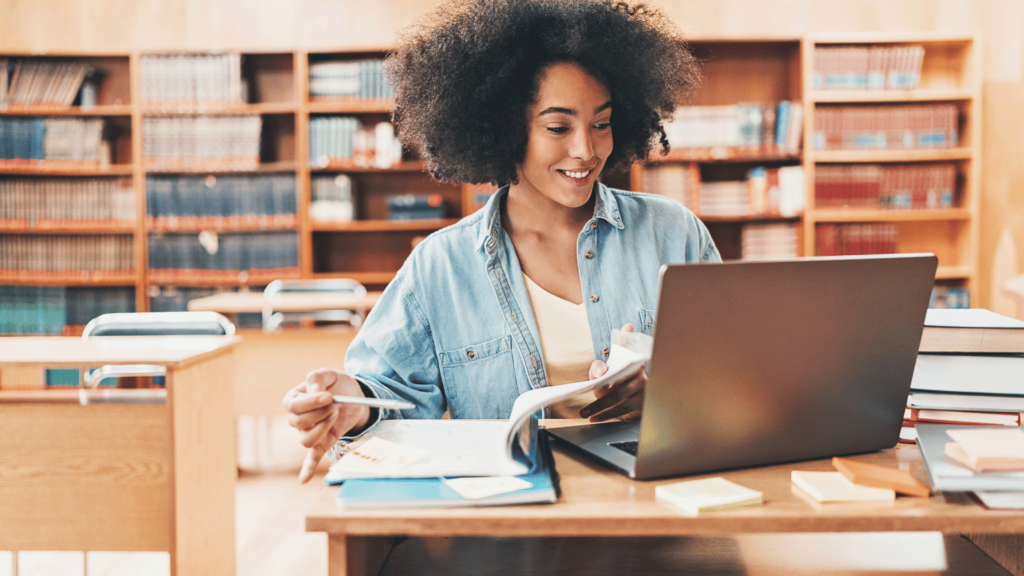
Master Award in Deep Learning
This unit provides a deep understanding of deep learning concepts and methodologies, covering applications in computer vision, NLP, and graph-based tasks. Learners will evaluate techniques, understand their limitations, and apply them to real-world problems, gaining the skills for deep learning research and practice.
Could transfer 20 credits and full tuition fee to the Master of Artificial Intelligence of SIMI Swiss and University Partners.
Learning Outcomes:
1. Understand the underlying theoretical concepts of modern deep learning methods.
-
1.1 Describe the fundamental principles of deep learning.
-
1.2 Explain the differences between supervised and unsupervised learning.
-
1.3 Critically analyse the role of gradient-based optimization in deep learning.
-
1.4 Evaluate the impact of network depth on model performance.
-
1.5 Discuss the theory of Variational Autoencoders (VAEs), Generative Adversarial Networks (GANs), and introduce Diffusion Models.
2. Be able to compare, characterise and quantitatively evaluate various deep learning approaches.
-
2.1 Describe and compare different architectures used in deep learning.
-
2.2 Critique the performance of CNNs in image analysis tasks.
-
2.3 Analyse the effectiveness of RNNs for sequential data processing.
-
2.4 Critically evaluate the performance of generative models in various tasks, including GANs and Diffusion Models.
-
2.5 Discuss transformer-based approaches to Large Language Models (LLMs).
3. Understand the limitations of deep learning.
-
3.1 Identify common challenges faced in deep learning.
-
3.2 Explain the concept of overfitting and strategies to mitigate it.
-
3.3 Analyse the ethical considerations associated with deep learning.
-
3.4 Critically evaluate the robustness and generalisation ability of deep learning models.
4. Be able to apply deep learning techniques to real-world problems in computer vision, speech, text analysis, and graph processing.
-
4.1 Implement CNNs for image classification tasks.
-
4.2 Apply RNNs to speech recognition and machine translation tasks.
-
4.3 Develop generative models for synthetic data generation.
-
4.4 Implement graph neural networks (GNNs) as a generalisation of CNN theory for graph-based data analysis.
Topics:
Introduction to Deep Learning
Course Coverage:
- Overview of Deep Learning
- Definition and Scope of Deep Learning
- Historical Context and Evolution of Neural Networks
- Biological Basis of Neural Networks: Non-linear activations and spiking neurons
- Importance of Deep Learning in Modern AI.
Learning Paradigms in AI
Course Coverage:
-
Supervised Learning
-
Basic Concepts and Examples
-
Applications in Classification and Regression
-
-
Unsupervised Learning
-
Fundamental Techniques (Clustering, Dimensionality Reduction)
-
Use Cases and Challenges
-
-
Generalization and Overfitting
-
Definitions and Differences
-
Implications for Model Training
-
Perceptrons and Neural Networks
Course Coverage:
- Perceptrons
- Single-Layer Perceptrons: Structure and Function
- Limitations and the XOR Problem
- Multi-Layer Perceptrons (MLP)
- Deep vs. Shallow Networks
- Advantages of Deep Architectures
Gradient-Based Optimization in Deep Learning
Course Coverage:
-
Stochastic Gradient Descent (SGD)
-
Concept and Mathematical Foundation
-
Variants of SGD (Momentum, Nesterov)
-
-
Backpropagation
-
Derivation and Role in Neural Network Training
-
Practical Implementation of Backpropagation
-
Introduction to Generative Models
Course Coverage:
-
Theory of Variational Autoencoders (VAE)
-
Mathematical Principles of VAEs
-
Applications of VAEs in Data Compression and Generation
-
-
Diffusion Models
-
Overview of Diffusion Models and Their Role in Generative Modeling
-
Comparison of Deep Learning Architectures
Course Coverage:
-
Perceptrons and Neural Networks:
-
Single-Layer vs. Multi-Layer Perceptrons
-
Non-Linear Activation Functions
-
-
Convolutional Neural Networks (CNNs)
-
Mathematical Foundations
-
Convolution Operations
-
Pooling Layers: Max and Average Pooling
-
-
CNN Architectures
-
Classic Architectures: LeNet, AlexNet, VGG
-
Advanced Architectures: ResNet, Inception
-
-
Recurrent Neural Networks (RNNs)
-
Understanding RNNs
-
Sequential Data Processing
-
Backpropagation Through Time (BPTT)
-
-
RNN Variants
-
Long Short-Term Memory (LSTM)
-
Gated Recurrent Units (GRU)
-
-
Applications of RNNs
-
Speech Recognition
-
Machine Translation.
-
-
-
Generative Models
-
Introduction to Generative Data Augmentation Techniques
-
Types: VAEs, GANs
-
Mathematical Principles of Generative Models
-
-
Generative Adversarial Networks (GANs)
-
Structure and Functioning of GANs
-
Challenges in Training GANs (Mode Collapse, Convergence Issues)
-
-
Applications of Generative Models
-
Image Generation and Enhancement
-
Data Augmentation Techniques
-
-
Incorporate Diffusion Models into Generative Models
-
Performance Evaluation of Deep Learning Models
-
Performance Metrics
-
Accuracy and Loss Metrics
-
Cross-Entropy, Mean Squared
-
Error
-
-
Evaluation Techniques
-
Confusion Matrix, Precision, Recall, F1-Score
-
ROC Curves and AUC
-
-
-
Case Studies in Deep Learning
-
Image Analysis
-
Performance of CNNs in Image Classification
-
Object Detection and Semantic Segmentation
-
-
Sequential Data Processing
-
RNNs in Time Series Forecasting
-
LSTMs in Language Modeling
-
-
Generative Networks
-
VAEs vs. GANs in Image Generation
-
Applications in Art and Content Creation
-
-
- Introduction to Transformer Models
- Image Transformers in LLMs
- Attention Mechanisms and Their Role in Natural Language Processing
Challenges in Deep Learning
- Data Requirements
- Large Datasets
- The Need for Big Data in Training Deep Models
- Challenges in Data Collection and Labeling
- Imbalanced Data
- Techniques to Handle Imbalanced Datasets
- Synthetic Data Generation (SMOTE, ADASYN)
- Large Datasets
- Computational Costs
- Hardware Requirements
- Role of GPUs and TPUs in Deep Learning
- Impact of Computational Power on Model Training
- Optimization Techniques
- Techniques to Reduce Computational Load
- Distributed Training and Model Parallelism
- Hardware Requirements
- Interpretability and Transparency
- The Black Box Problem
- Lack of Transparency in Deep Models
- Importance of Model Interpretability
- Techniques for Interpretability
- Saliency Maps, Grad-CAM, LIME
- Explainable AI (XAI) Approaches
- The Black Box Problem
Overfitting and Generalization in Deep Learning
- Overfitting in Deep Models
- Causes of Overfitting
- High Model Complexity
- Insufficient Training Data
- Strategies to Mitigate Overfitting
- Regularization Techniques: L1, L2, Dropout
- Early Stopping and Cross-Validation
- Causes of Overfitting
- Generalization Challenges
- Transfer Learning
- Concepts and Benefits
- Pre-Trained Models and Fine-Tuning
- Adversarial Examples
- Impact on Model Robustness
- Techniques to Improve Robustness
- Transfer Learning
- Ethical Considerations in Deep Learning
- Bias in AI Models
- Sources and Implications of Bias
- Strategies to Mitigate Bias
- Privacy Concerns
- Data Privacy in AI Applications
- Techniques to Ensure Data Security
- Fairness and Accountability
- Ensuring Ethical AI Practices
- Case Studies in Ethical AI Implementation
- Bias in AI Models
Computer Vision with Deep Learning
- Introduction to Attention Mechanisms
- Convolutional Neural Networks (CNNs) Transfer Learning in CNNs
Speech and Language Processing with RNNs
-
Applications in Real-Time Translation Systems
Generative Models for Synthetic Data Creation
-
Diffusion Models for Generative Data Augmentation
Graph Neural Networks (GNNs)
-
Introduce GNNs as a Generalization of CNN Theory
-
Applications in Medicine and Biology
Indicative reading list
Core texts:
-
Goodfellow, I., Bengio, Y., & Courville, A. (2016). Deep Learning. MIT Press.
-
Vaswani, A., et al. (2017). Attention Is All You Need. NeurIPS.
-
Zhang, Y., et al. (2019). Graph Neural Networks: A Review of Methods and Applications. arXiv: 1812.08434
Additional reading:
-
MIT Deep Learning for Self-Driving Cars: https://deeplearning.mit.edu/
-
NVIDIA Deep Learning Institute: www.nvidia.com/dli
Entry requirements
To enroll The Master Award, the learner must possess:
- Graduated with a Bachelor’s degree from an accredited university or achieved a Level 6 Diploma according to the European Qualifications
- For a degree from non-recognized universities; The learner should have followed Accreditation of Prior Experiential Learning for Qualifications (APEL.Q) policy of SIMI and/or University Partners.
- Learners must be over 21 years old.
The SIMI Swiss reserves the highest decision-making power for admission whether to accept or not accept after a specific review of each candidate’s profile to ensure that they can comprehend and gain benefits when participating. For the fake university or diploma mills, University Partners shall not be accepted.
English requirements
If a learner is not from a predominantly English-speaking country, proof of English language proficiency must be provided.
- Common European Framework of Reference (CEFR) level B2 or equivalent
- Or A minimum TOEFL score of 101 or IELTS 6.5; Reading and Writing must be at 6.5 or equivalent
After graduating with Master Award, students receive all certified documents from the SIMI Swiss.
Certified Documents:
- e-Certificate from the Swiss Information and Management Institute (SIMI Swiss).
- Hard copy certificate from the Swiss Information and Management Institute (SIMI Swiss) – Optional.
- Accreditation of Prior Experiential Learning for Qualifications (APEL.Q) certified from SIMI Swiss for credit and tuition fee transfer.
Because the program is accredited and recognized, students can easily use certified in the working environment and have many opportunities for career advancement. In addition, in case if you want to study for a SIMI degree or university partner degree, students can convert all credits and the full paid tuition fee when participating in the program University Partners.
The SIMI Swiss’ Master Award means:
SIMI Swiss Master Award is the award at the master level and is equivalent to:
- Level 7 certificate of Regulated Qualification Framework (RQF) of UK
- Level 10 certificate of Scottish Credit and Qualifications Framework (SCQF)
- Level 7 certificate of Credit and Qualifications Framework (CQFW)
- Level 7 certificate of European Qualifications Framework (EQF)
- Level 9 certificates of the Australian Qualifications Framework (AQF)
- Level 7 certificate of ASEAN Qualifications Reference Framework (AQRF)
- Level 9 certificate of the African Continental Qualifications Framework (ACQF)
Students can convert all credits and the full tuition fee when participating in the SIMI Swiss and/or University Partners academic programs if they want to study for an academic degree.
Credits transfer:
Learners can accumulate 20 credits from the Master Award program when participating in the Master of Artificial Intelligence. Please see the credit transfer policy HERE
Tuition fee transfer:
When participating in the Master of Artificial Intelligence program, students who have graduated 1 Master Award will receive a discount of full tuition fee which you paid. Please see the tuition fee transfer HERE
The SIMI Swiss micro-credential program allows for the transfer of credits and tuition fees into full degree programs from SIMI Swiss and/or its university partners. SIMI Swiss reserves the right to limit admissions once the number of students exceeds the quotas.
Apply Policy:
- To participate in the SIMI Swiss micro-credential program, students need to meet the entry criteria corresponding to each level. Please see the “Entry” tab for more details.
- SIMI Swiss will not accept applicants if their entry qualifications are from diploma mill universities or schools/universities that are not accredited.
- For Master Award programs, if an entry bachelor is unavailable, students must demonstrate a minimum of 5 years of work experience in the relevant field. Please note that a bachelor’s degree is required for the Master’s program at SIMI Swiss and University Partners so that you could study Master Award but could not move to the Master’s program of SIMI and University Partners.
- English is not a mandatory entry requirement for short course programs, but candidates need to ensure that English is used in reading documents, listening to lectures, and doing assignments. Candidates should note that English is a mandatory requirement when switching to an academic program at SIMI Swiss and University Partners.
Apply Process:
- Choose the program that suits your requirements. Note that applicants without a university degree will not be able to participate in the program at Master’s level, and applicants without a Master’s degree will not be able to participate in the program at the Doctoral level.
- Email your application to support@simiswiss.ch with all the required documents. You could download the application form here.
- Our admission department will contact you and guide you through further processes if the registration documents need to be supplemented.
- SIMI Swiss will issue the Letter of Acceptant (LOA). You wil proceed to the next steps according to the instructions and pay tuition fee.
- SIMI Swiss will issue a student confirmation letter, login account to the e-learning system and related documents.
- You have become an official SIMI Swiss student and enjoy your study journey.
The SIMI Swiss micro-credential program is fully online, allowing you to study anytime, anywhere. You have the option to attend live classes with SIMI Swiss. The final exam will be uploaded to the system and evaluated by the academic panel of SIMI Swiss. Students must submit assignments on time; failure to do so will result in the student being considered to have discontinued the program.
Pricing Plans
Take advantage of one of our non-profit professional certified programs with favorable terms for your personal growing carreers.
- Live Class (Option)
- Full online videos
- e-Books
- Self study contents
- Online tutor videos
- Assignment guide
- e-Certificate
- Hard copy certificate
- Accreditation of Prior Experiential Learning for Qualifications (APEL.Q) certified from University Partners for credit and tuition fee transfer
- Accreditation & Recognition certified from University Partners
- Deliver hard copy certificate and all certified documents to your home
- Transfer full credits & tuition fees to equivalent academic programs
- Get more support tuition fees and scholarships when becoming University Partners' international students
- (*) In the event that you receive a scholarship or discount, the fee you should transfer is the amount you actually paid.
SWISS MICRO CREDENTIAL
Contact us
If you interested this micro credential course, please feel free to contact with us! Please note that this program is a not for profit and learning with full online model.